Precision Subclassification of Type 2 Diabetes
Nature Communications Medicine
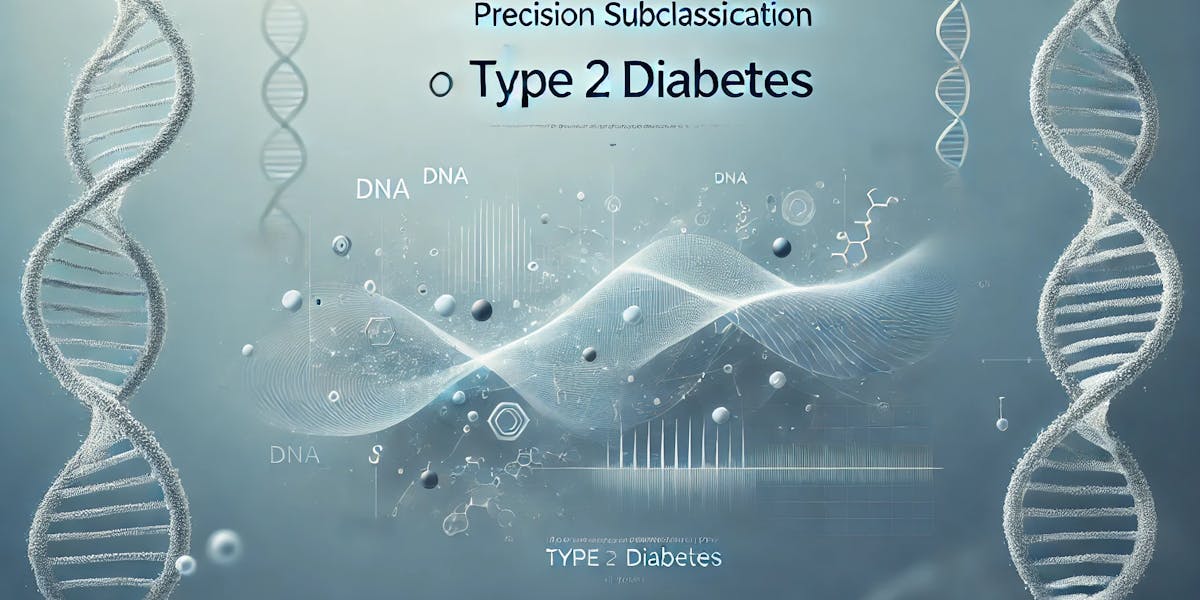
Summary
This systematic review analyzed studies on type 2 diabetes subclassification using both simple clinical measures and complex machine learning approaches. While many classification models exist, most lack replication and clinical applicability. More research is needed to validate and implement precision diabetes care.
Study Design
Interventions
Study Type
Outcomes
Duration and Size
Study Population
Age Range
Sex
Geography
Other Criteria
Methodology
This study systematically reviewed literature from PubMed and Embase, focusing on both simple (clinical characteristics) and complex (machine learning, omics) subclassification methods for type 2 diabetes. The quality of studies was evaluated using GRADE criteria, and results were categorized based on reproducibility and clinical impact. While multiple classification models were identified, few demonstrated consistent clinical applicability or outcome benefits.
Interventions
The study categorized type 2 diabetes into subtypes based on clinical variables (BMI, glucose levels, lipids) and computational models (machine learning, genomic clustering). It compared outcomes such as disease progression, medication response, and complication risks across these subtypes.
Key Findings
The study found that while machine learning and genomic clustering provide promising subclassifications for type 2 diabetes, few have been replicated or tested in clinical trials. Simple classification methods remain more accessible but lack precision. Future research should focus on testing these subtypes in diverse populations and assessing their impact on personalized treatment strategies.
Comparison with other Studies
The study "Precision Subclassification of Type 2 Diabetes: A Systematic Review" (DOI: 10.1038/s43856-023-00360-3) aligns with several other significant studies investigating Type 2 Diabetes (T2D) subtypes. One such study, "Subtypes of Type 2 Diabetes Determined From Clinical Parameters", identified four distinct T2D subtypes—Severe Insulin-Deficient Diabetes (SIDD), Severe Insulin-Resistant Diabetes (SIRD), Mild Obesity-Related Diabetes (MOD), and Mild Age-Related Diabetes (MARD)—using clinical data clustering based on BMI, HbA1c, and insulin resistance indices, demonstrating variations in subtype prevalence across populations (diabetesjournals.org). Another study, "Novel Subgroups of Type 2 Diabetes Display Different Epigenetic Patterns", explored DNA methylation differences among these subtypes, revealing distinct epigenetic signatures that suggest diverse etiologies and potential for tailored treatments (pmc.ncbi.nlm.nih.gov). The research "Identifying Subtypes of Type 2 Diabetes Mellitus with Machine Learning" applied machine learning techniques to classify T2D into Metabolic, Early Onset, Late Onset, and Cardiometabolic subtypes, with significant differences in 5-year mortality, hospitalization rates, and chronic disease incidence, reinforcing the potential of AI-driven precision medicine (drc.bmj.com). Similarly, "Etiologies Underlying Subtypes of Long-Standing Type 2 Diabetes" mapped these subtypes to those identified in newly diagnosed patients, confirming their reproducibility and the potential for subtype-specific interventions across the disease course (pmc.ncbi.nlm.nih.gov). These studies collectively highlight the increasing consensus on T2D subclassification, reinforcing the importance of personalized treatment strategies that address specific pathophysiological mechanisms. Consistency across research findings strengthens the argument for integrating subtype-based management into clinical practice, potentially improving outcomes by moving beyond the traditional one-size-fits-all approach to diabetes care.
Journal Reference
Precision Subclassification of Type 2 Diabetes: A Systematic Review Author(s). Nat Commun Med. 2023;3(1):Article 360. doi:10.1038/s43856-023-00360-3.
Related and Discussions
Study Reveals Genetic Clusters That May Explain Differences in Type 2 Diabetes Risk
Prevención de la diabetes tipo 2: una revisión sistemática y metaanálisis de diferentes estrategias de intervención
Revisión sistemática y metaanálisis actualizados sobre los determinantes sociales de la diabetes y los factores de riesgo conexos en el Caribe
A wealth of #diabetes subtype-specific information is emerging from recent studies.
Machine learning and #T2D #diabetes subtypes: On the road to improving clinical outcomes?
New @StanfordMed research shows #AI's potential to predict & identify subtypes of #Type2Diabetes.
In this study, @mshimabukuro38 and colleagues have developed a machine learning-based model that can reproducibly predict subtypes of type 2 diabetes.
Discover Type 2 Diabetes subtypes and treatment insights in this article by Lili Grieco-St-Pierre, PhD student, and Jennifer Bruin, Associate Professor.
Stay informed. Stay ahead.
Subscribe now for the latest breakthroughs, expert insights, and cutting-edge updates in diabetes care—delivered straight to your inbox.