Chatbots for Diabetes Self-Management
Journal of Medical Internet Research
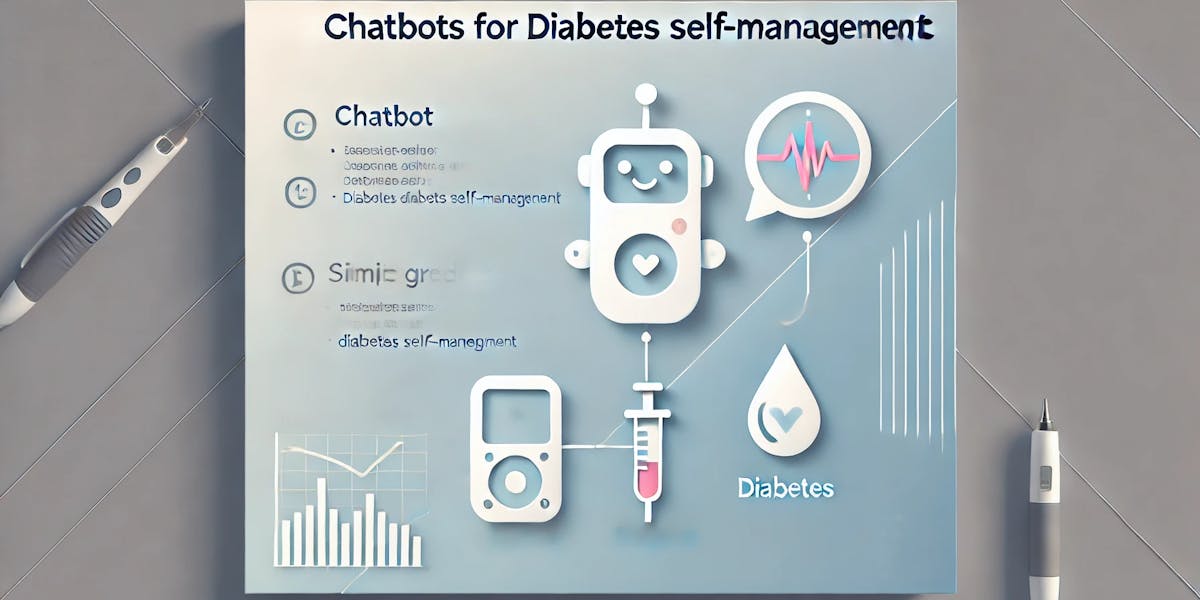
Summary
This study provides a systematic review and meta-analysis of chatbot interventions designed to support diabetes self-management. It analyzed 25 studies from 14 countries published up to January 1, 2023, which included system design, pilot, and intervention studies. The chatbots commonly focused on self-management components such as diet, exercise, medication adherence, glucose monitoring, and management of complications. The interventions varied in format, offering text or voice interfaces with content drawn from reputable sources including diabetes guidelines. Most studies showed high user acceptance, particularly for personalized and interactive chatbot designs. The review used quality assessment tools and data synthesis methods, including the PRISMA framework and meta-analysis models, to evaluate the effectiveness of chatbots. Meta-analysis results revealed a statistically significant reduction in HbA1c (mean difference 0.30, 95% CI 0.04-0.55; P=.02) but not in weight (mean difference 1.41, 95% CI –2.29 to 5.11; P=.46). The review emphasized that most studies were of low to moderate quality, lacking rigorous RCT designs. Furthermore, the study identified gaps including limited research in low-income countries, underuse of mixed methods, and insufficient application of theoretical frameworks. The authors recommend future studies adopt more robust designs, utilize mixed-method approaches, and focus on personalized, scalable, and privacy-respecting chatbot tools for diabetes care.
Study Design
Interventions
Study Type
Outcomes
Duration and Size
Study Population
Age Range
Sex
Geography
Other Criteria
Methodology
This study followed the PRISMA guidelines for systematic reviews and performed a structured search in PubMed and Web of Science through January 1, 2023. Two reviewers independently screened titles, abstracts, and full texts using predefined inclusion/exclusion criteria. A quality scoring tool based on Agency for Healthcare Research and Quality guidelines was used to rate methodological quality. The study focused on chatbot interventions offering educational and behavioral support in diabetes care. Data were extracted into a standardized form capturing study type, design, intervention features, user interaction type, and effectiveness criteria. Conflicts in screening or scoring were resolved via consensus.
Meta-analyses were performed using RevMan 5.3 for studies reporting pre-post designs, focusing on HbA1c and body weight changes. Homogeneity of data was assessed using I² and P values, with fixed-effect models used when heterogeneity was low. A funnel plot was used to assess publication bias. Results were synthesized to determine the efficacy of chatbot interventions and examine patterns by country, chatbot design, and study approach. The methodology also reviewed how chatbots used AI, content sources, and interaction modes (text, button, voice), providing a rich contextual understanding of chatbot applications in diabetes care.
Interventions
The interventions reviewed included chatbot platforms delivering diabetes self-management education and behavioral coaching. Chatbots were accessed through text or voice interfaces on mobile or web platforms. The chatbots provided personalized advice and reminders regarding glucose monitoring, medication adherence, diet, exercise, and complications prevention. Many used motivational interviewing techniques, gamified features, and behavior change theories to improve adherence. Some allowed for data input by patients and returned feedback or connected users to health professionals. Features like educational modules, interactive Q&A, and virtual rewards were common. Only a minority addressed mental health needs or supported multilingual populations.
Key Findings
Chatbots significantly improved HbA1c levels (mean diff. = 0.30, P = .02) but showed no significant effect on weight. Most studies confirmed high user satisfaction and feasibility. The educational and engagement strategies, especially those grounded in theoretical frameworks like the Behavior Change Wheel, were positively received. Chatbots were found useful in delivering tailored advice, reminders, and support outside clinical settings. However, evidence was mostly from small-scale or pilot studies, lacking robust RCTs. Studies also noted the need for personalized content, data privacy measures, and broader accessibility to diverse populations.
Comparison with other Studies
Compared to prior literature on digital interventions for diabetes, this review confirms the utility of chatbots in improving glycemic control. Unlike general mobile health tools, chatbots provide interactive, user-driven experiences. Prior studies on AI in diabetes management often emphasized monitoring and data capture; this study highlights chatbots’ role in behavior change and education. The review also aligns with meta-analyses on other chronic diseases showing chatbots improve adherence and outcomes. However, it differs by identifying the limited evidence base specific to diabetes-focused chatbots, and the underutilization of mixed methods or RCTs to evaluate these technologies.
Journal Reference
Wu Y, Zhang J, Ge P, et al. Application of Chatbots to Help Patients Self-Manage Diabetes: Systematic Review and Meta-Analysis. J Med Internet Res. 2024;26:e60380. doi:10.2196/60380
© 2025 deDiabetes. Licensed under CC BY (Attribution)
Stay informed. Stay ahead.
Subscribe now for the latest breakthroughs, expert insights, and cutting-edge updates in diabetes care—delivered straight to your inbox.